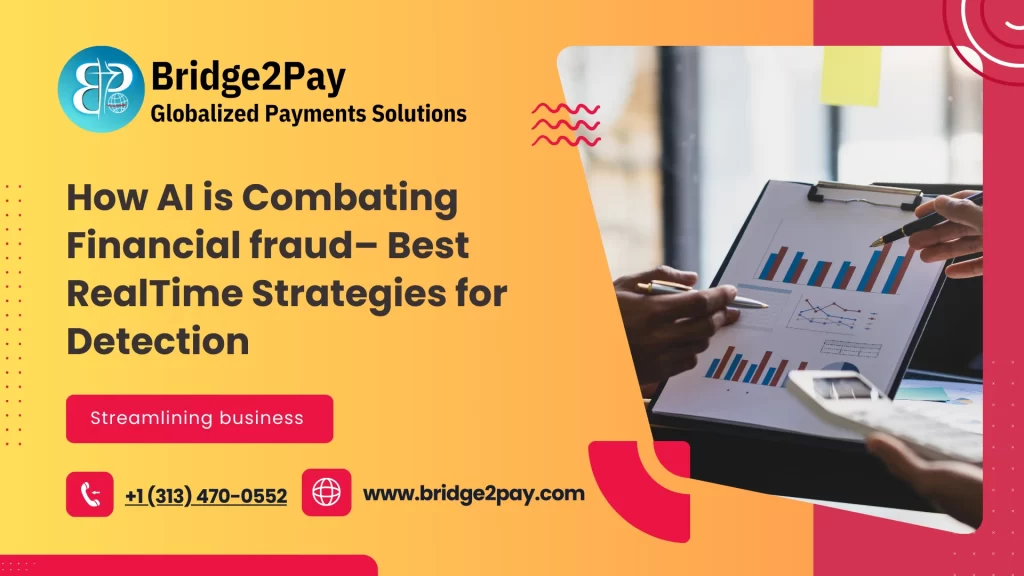
Introduction
The threat of financial fraud continues to grow in the digital age, as cybercriminals develop increasingly advanced techniques to exploit weaknesses in banking, e-commerce, and financial services. Conventional security measures based on fixed rules are no longer sufficient to counteract these evolving threats. Artificial Intelligence (AI) is reshaping fraud detection and prevention by processing vast amounts of data in real time, recognizing patterns, and identifying suspicious behavior with unparalleled efficiency and precision.
This article explores the transformative role of AI in Financial fraud detection, the core strategies it employs, and the future of AI-driven fraud prevention.
The Escalating Challenge of Financial Fraud
With the rise of digital banking, online shopping, and contactless payments, the risks of financial fraud have significantly increased. Common types of fraudulent activity include:
- Identity Theft – Criminals steal personal data to impersonate victims and conduct unauthorized financial activities.
- Credit Card Fraud – Stolen card details are used to carry out fraudulent purchases.
- Account Takeover – Hackers gain control of banking accounts to withdraw funds or initiate unauthorized transactions.
- Phishing Attacks – Deceptive emails, messages, or fake websites trick users into revealing sensitive financial information.
- Money Laundering – Criminals disguise the origins of illicit funds by moving them through multiple financial transactions.
- Synthetic Identity Fraud – Fraudsters construct fictitious identities using a combination of real and fabricated details to open accounts and obtain credit.
- Insider Threats – Employees within financial institutions misuse their access to commit fraud or leak sensitive data.
AI-powered Financial fraud detection systems combat these threats in real time by utilizing machine learning, predictive analytics, and behavioral pattern analysis to proactively identify and mitigate fraudulent activity before it results in financial losses.
AI Strategies for Real-Time Fraud Prevention
1. Machine Learning for Anomaly Detection
AI-driven Financial frauddetection systems leverage machine learning algorithms to assess past transaction data and determine typical spending behaviors. If an anomaly occurs—such as an unusually high transaction amount or an unfamiliar geographical location—AI flags it for further investigation.
2. Behavioral Biometrics and Identity Authentication
AI enhances security through biometric authentication, including fingerprint scanning, facial recognition, and voice authentication. Additionally, AI assesses behavioral patterns, such as typing speed and mouse movements, to detect unauthorized account access attempts.
3. Continuous Transaction Monitoring
AI provides ongoing surveillance of financial transactions, identifying red flags like rapid transactions from different locations, large withdrawals, or deviations from a user’s normal spending patterns. This enables financial institutions to block fraudulent transactions in real time.
4. Natural Language Processing (NLP) to Counter Phishing
Phishing scams often contain linguistic indicators that AI-powered NLP tools can detect. By analyzing email content, AI helps identify fraudulent messages and warns users before they become victims.
5. Network Analysis for Money Laundering Detection
AI uses network analysis to track the movement of funds across different accounts, uncovering suspicious patterns indicative of money laundering schemes.
6. Automated Fraud Case Management
AI assists financial institutions by categorizing flagged transactions based on risk levels. This prioritization ensures that analysts can focus their attention on high-risk cases first.
7. AI-Based Risk Assessment
AI assigns risk scores to financial transactions based on variables such as user location, transaction history, and device usage. Transactions exceeding a risk threshold are flagged for review or blocked automatically.
8. Deep Learning for Enhanced Fraud Detection
Deep learning algorithms analyze extensive datasets to uncover sophisticated fraud patterns that conventional Financial fraud detection systems might overlook. AI continually refines its detection abilities to counteract emerging fraud tactics.
AI’s Contribution to Strengthening Cybersecurity
1. Dynamic Fraud Prevention Systems
Unlike static, rule-based fraud detection methods, AI-powered systems continuously evolve to counter new fraud techniques. By learning from new datasets, AI strengthens its fraud detection over time.
2. Minimizing False Positives
Distinguishing genuine transactions from fraudulent ones remains a challenge. AI minimizes false alarms by refining its models to improve accuracy, ensuring that legitimate transactions aren’t unnecessarily declined.
3. AI-Enabled Chatbots for Fraud Prevention
institutions employ AI-driven chatbots to engage with customers in real time. These chatbots alert users to suspicious activity on their accounts and request verification when necessary.
4. Collaborative AI Networks
AI-poweredFinancial fraud detection systems share anonymized fraud data across financial institutions, improving fraud detection capabilities by recognizing suspicious patterns on a wider scale.
5. AI in Cybersecurity for Financial Protection
AI enhances cybersecurity measures by detecting malware, monitoring dark web activities, and identifying system vulnerabilities to prevent potential breaches leading to financial fraud.
The Future of AI in Financial Fraud Detection
AI’s role in fraud prevention is set to become even more advanced, with future innovations including:
- AI-Powered Blockchain Analysis – AI will enhance blockchain transaction tracking to detect fraud in decentralized finance (DeFi) ecosystems.
- Advanced Deep Learning Algorithms – AI will continue to refine deep learning models to detect increasingly complex fraud techniques.
- AI and Regulatory Collaboration – AI-powered fraud detection will integrate with regulatory bodies to ensure compliance with financial security laws.
- Explainable AI (XAI) for Transparency – AI fraud detection systems will incorporate explainable AI to provide clear justifications for flagged transactions, improving regulatory compliance and user trust.
- Quantum AI for Fraud Prevention – As quantum computing advances, AI will be able to process vast amounts of financial transaction data in real time, enabling even faster and more accurate fraud detection.
- AI-Generated Fraud Intelligence Reports – AI will automate fraud intelligence reporting, enabling financial institutions to proactively identify threats and implement preventive measures.
- Enhanced AI-Powered Identity Security – AI will improve identity verification by cross-referencing credentials across multiple secure databases, reducing the risk of identity fraud.
- AI Integration with IoT Devices – AI will collaborate with Internet of Things (IoT) devices, such as smart payment systems and connected banking solutions, to detect and prevent fraud at the transaction point.
How AI is Tackling Financial Fraud – Real-Time Detection Strategies
The risk of Financial fraud is growing rapidly in the digital era, with cybercriminals continuously developing sophisticated techniques to exploit vulnerabilities in banking, e-commerce, and financial services. Traditional security measures that rely on fixed rules are proving inadequate against these evolving threats. Artificial Intelligence (AI) is transforming fraud detection and prevention by processing massive amounts of data in real time, identifying patterns, and detecting suspicious activities with unmatched efficiency and accuracy.
This article delves into AI’s transformative role in fraud detection, its core strategies, and the future of AI-powered fFinancial fraud prevention, enriched with case studies and visual insights.
The Rising Threat of Financial Fraud
With the expansion of digital banking, e-commerce, and contactless transactions, financial fraud has escalated significantly. The most common forms of fraudulent activities include:
- Identity Theft – Criminals acquire personal data to impersonate individuals and carry out unauthorized financial transactions.
- Credit Card Fraud – Stolen card details are used to execute fraudulent purchases.
- Account Takeover – Hackers infiltrate banking accounts to withdraw funds or initiate unauthorized payments.
- Phishing Attacks – Fraudsters use deceptive emails, messages, or fake websites to extract sensitive financial data from users.
- Money Laundering – Criminals obscure the origins of illicit funds by routing them through multiple financial channels.
- Synthetic Identity Fraud – Fraudsters create false identities using a combination of real and fabricated information to open accounts and secure credit.
- Insider Threats – Employees within financial institutions exploit their access to commit fraud or leak confidential information.
AI-drivenFinancial fraud detection systems address these threats in real time through machine learning, predictive analytics, and behavioral analysis, proactively identifying and mitigating fraudulent activities before they cause financial losses.
AI-Driven Strategies for Real-Time Fraud Prevention
1. Machine Learning for Identifying Anomalies
AI-powered Fianancial fraud detection leverages machine learning algorithms to examine past transaction data and determine typical spending habits. When anomalies arise—such as an unusually high-value transaction or an unfamiliar location—AI flags them for further scrutiny.
2. Behavioral Biometrics and Identity Verification
AI enhances security by incorporating biometric authentication methods, including fingerprint scanning, facial recognition, and voice verification. Furthermore, AI evaluates behavioral traits like keystroke dynamics and mouse movements to identify unauthorized access attempts.
3. Continuous Monitoring of Transactions
AI ensures constant oversight of financial transactions, detecting warning signs such as rapid transactions from different locations, large withdrawals, or deviations from usual spending habits. This enables financial institutions to block fraudulent activities in real time.
4. Natural Language Processing (NLP) for Phishing Prevention
AI-powered NLP tools detect linguistic clues in phishing scams. By scanning email content, AI helps identify fraudulent messages and warns users before they fall victim.
5. Network Analysis for Money Laundering Prevention
AI employs network analysis to trace fund movements across multiple accounts, uncovering suspicious patterns indicative of money laundering.
6. Automated Fraud Case Prioritization
AI streamlines Financial fraud detection by classifying flagged transactions based on risk levels, allowing analysts to concentrate on high-risk cases first.
7. AI-Powered Risk Evaluation
AI assigns risk scores to transactions based on variables such as user location, transaction history, and device usage. Transactions exceeding a risk threshold are automatically flagged for review or blocked.
8. Advanced Deep Learning for Fraud Detection
Deep learning models process vast datasets to detect complex fraud patterns that traditional methods might overlook. AI continuously refines its detection capabilities to counter emerging Financial fraud tactics.
Case Studies
Case Study 1: AI Preventing Credit Card Fraud in a Leading Bank
A prominent global bank deployed an AI-based fraud detection system to monitor transaction patterns. The system analyzed millions of transactions daily, successfully reducing false positives by 40% and detecting 25% more fraudulent activities than conventional rule-based systems within six months.
Case Study 2: AI Combatting Money Laundering
A financial firm specializing in international transactions incorporated AI-powered network analysis to detect money laundering. The AI system identified irregular money flows and flagged suspicious accounts, preventing over $200 million in fraudulent transactions within a year.
Case Study 3: AI Enhancing Fraud Detection in E-Commerce
An e-commerce platform adopted AI-driven behavioral biometrics and NLP tools to detect fraudulent purchases. By analyzing user interactions, device fingerprinting, and payment behaviors, the platform successfully reduced chargeback fraud by 35% and bolstered customer trust.
AI’s Role in Strengthening Cybersecurity
1. Adaptive Fraud Prevention Systems
Unlike static, rule-based systems, AI-powered fraud detection evolves continuously to counter emerging Financial fraud tactics. By learning from new data, AI enhances its fraud detection capabilities over time.
2. Reducing False Positives
Differentiating between legitimate and fraudulent transactions remains a challenge. AI minimizes false alarms by refining its models, ensuring that genuine transactions are not unnecessarily declined.
3. AI-Powered Chatbots for Fraud Alerts
Financial institutions deploy AI-driven chatbots for real-time customer engagement. These chatbots notify users about suspicious account activities and request verification when necessary.
4. Collaborative AI Networks
AI-based fraud detection systems share anonymized fraud data across financial institutions, improving fraud detection capabilities through broader pattern recognition.
5. AI in Cybersecurity to Safeguard Financial Systems
AI strengthens cybersecurity by identifying malware, monitoring dark web activities, and detecting vulnerabilities that could lead to financial fraud.
Visual Insights
Comparison of AI-Based and Traditional Fraud Detection
(A bar chart contrasting AI-driven detection with conventional fraud detection approaches.)
Reduction in False Positives with AI Implementation
(A line graph illustrating the decrease in false alerts following AI integration.)
AI’s Improvement in Fraud Detection Efficiency Over Time
(A graph showing AI’s increasing accuracy in fraud detection.)
The Future of AI in Financial Fraud Prevention
AI’s role in fraud detection will continue to advance with the following innovations:
- AI-Enhanced Blockchain Analysis – AI will optimize blockchain transaction tracking to prevent fraud in decentralized finance (DeFi) ecosystems.
- More Sophisticated Deep Learning Algorithms – AI will further refine deep learning models to detect intricate fraud tactics.
- AI and Regulatory Compliance – AI-powered fraud detection will integrate with financial regulators to ensure compliance with security laws.
- Explainable AI (XAI) for Greater Transparency – AI-driven fraud detection will incorporate explainable AI to justify flagged transactions, enhancing compliance and user trust.
- Quantum AI for Fraud Mitigation – Advancements in quantum computing will enable AI to analyze financial transactions in real time with even greater accuracy.
AI’s Role in Strengthening Cybersecurity
- Adaptive Fraud Prevention Systems
Unlike static, rule-based methods, AI-driven fraud detection evolves continuously to counter new fraud techniques. AI improves its fraud detection efficiency by learning from fresh datasets over time.
- Reducing False Positives
Distinguishing between legitimate and fraudulent transactions is a challenge. AI minimizes false alerts by refining its models, ensuring that genuine transactions are not unnecessarily blocked.
- AI-Powered Chatbots for Fraud Alerts
Financial institutions deploy AI-driven chatbots for real-time customer engagement. These chatbots notify users about suspicious account activity and request verification when necessary.
- Collaborative AI Networks
AI-powered fraud detection systems share anonymized fraud data across financial institutions, enhancing fraud prevention by recognizing patterns on a broader scale.
- AI in Cybersecurity for Financial Protection
AI strengthens cybersecurity by identifying malware, monitoring dark web activities, and detecting system vulnerabilities that could lead to financial fraud.
Visual Insights
- Comparison of AI-Based and Traditional Fraud Detection
- (A bar chart comparing AI-driven detection with conventional fraud detection approaches.)
- Reduction in False Positives with AI Implementation
- (A line graph illustrating the decrease in false alerts after AI integration.)
- AI’s Increasing Fraud Detection Efficiency Over Time
- (A graph demonstrating AI’s growing accuracy in fraud detection.)
The Future of AI in Financial Fraud Prevention
AI’s role in fraud detection will continue to evolve with innovations such as:
- AI-Enhanced Blockchain Analysis – AI will optimize blockchain transaction tracking to prevent fraud in decentralized finance (DeFi) ecosystems.
- Advanced Deep Learning Models – AI will refine deep learning algorithms to detect increasingly complex fraud techniques.
- AI and Regulatory Compliance – AI-powered fraud detection will integrate with regulators to ensure compliance with financial security laws.
- Explainable AI (XAI) for Transparency – AI will provide clear justifications for flagged transactions, improving compliance and user trust.
- Quantum AI for Fraud Prevention – Quantum computing advancements will enable AI to process vast amounts of transaction data in real time with greater accuracy.
- AI-Generated Fraud Intelligence Reports – AI will automate fraud intelligence reporting, allowing financial institutions to identify threats proactively.
- Enhanced AI-Powered Identity Security – AI will enhance identity verification by cross-referencing credentials across multiple secure databases.
- AI Integration with IoT Devices – AI will collaborate with IoT devices, such as smart payment systems and connected banking solutions, to detect and prevent fraud at the transaction point.
Conclusion
AI is revolutionizing financial fraud detection by analyzing extensive datasets, identifying anomalies, and responding to threats instantly. By harnessing machine learning, behavioral biometrics, and real-time transaction monitoring, AI strengthens fraud prevention and fortifies cybersecurity within the financial sector. As AI continues to evolve, its role in safeguarding digital transactions and reducing financial fraud globally will become even more significant.
Financial institutions, businesses, and individuals must proactively adopt AI-driven fraud detection solutions to outpace cybercriminals. While AI significantly mitigates fraud risks, ongoing advancements in AI technology, compliance measures, and cybersecurity protocols will be crucial in building a more secure financial landscape for the future.